Thought Leader Event
Q-Park and Erasmus University Rotterdam held a Thought Leader Event at Lumière Cinema Maastricht. The jointly organised Thought Leader Event focused on what parking experts and students have to say about parking and mobility.
The event began with Q-Park CEO Frank De Moor presenting his thoughts on A bright future for parking. Erasmus University Rotterdam researcher Giuliano Mingardo then gave a presentation about Future Trends in Mobility.
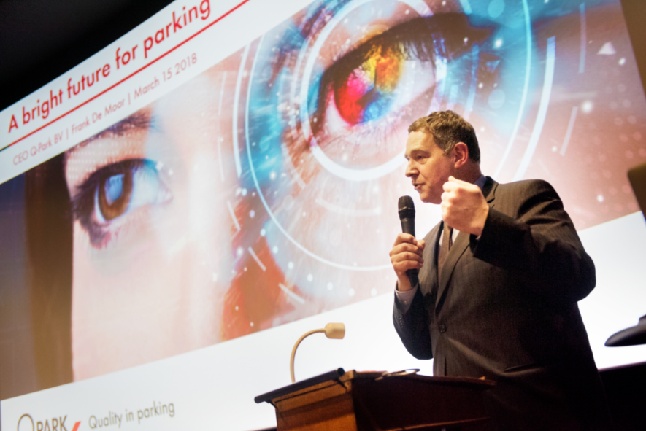
Frank De Moor (CEO Q-Park BV) - Presenting A bright future for parking.
Next on the agenda was the presentation of the Ward Vleugels Q-Park Thesis Award, for the master thesis with the most academic and socio-economic relevance for the parking sector.
Download the combined presentations here.
Ward Vleugels Q-Park Thesis Award 2017
This year, seven theses were submitted from five universities: Delft, Amsterdam, Rotterdam, Eindhoven and Hasselt. A jury of academics from various universities selected three finalists from all the entries based on the economics of the submissions:
The effects of private automated vehicles on drivers' parking location choice, by Daphne van den Hurk (Technical University (TU) Delft)
The social costs of on-street parking-searching, policy and unpriced externalities, by Michael Mclvor (Vrij Universiteit (VU) Amsterdam)
Know before you go: predicting parking space occupancy by exploiting publicly accessible data, by Robert Boer (Erasmus University Rotterdam (EUR))
And the winner is…
Based on the originality of the topic and the depth of the analysis, a panel of parking experts from the industry have selected both Daphne and Robert as the winners. The cheques for the ex aequo winners of EUR 2,500 each and the runner-up Michael McIvor of EUR 500 were presented by Ward Vleugels, Founder of Q-Park.
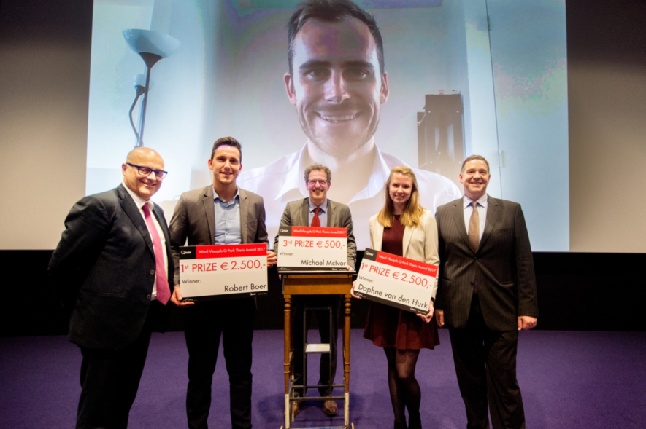
Left to right: Ward Vleugels, Robert Boer, Michael McIvor (on-screen, and cheque held by Theo Thuis - Managing Director Innovations at Q-Park), Daphne van den Hurk, and Frank De Moor.
Summary of the winning theses;
The effects of private automated vehicles on drivers' parking location choice, by Daphne van den Hurk (Technical University (TU) Delft)
The objective of this research is to find the importance of different factors and constraints that could influence drivers’ parking location choice for a future situation in which private highly AVs will become available for passenger transport. The two considered parking locations are 1) parking in the inner city (PIC) and 2) parking at the edge of the city (PEC), both at off-street parking facilities.
From the results of the hypothetical choice situations, it can be concluded that in general PIC is preferred over PEC. The ‘parking cost’, the ‘risk of extra waiting time’ and the ‘risk of parking fee’ have a negative influence on drivers’ parking location choice. ‘Personnel surveillance’ has a positive influence on drivers’ parking location choice.
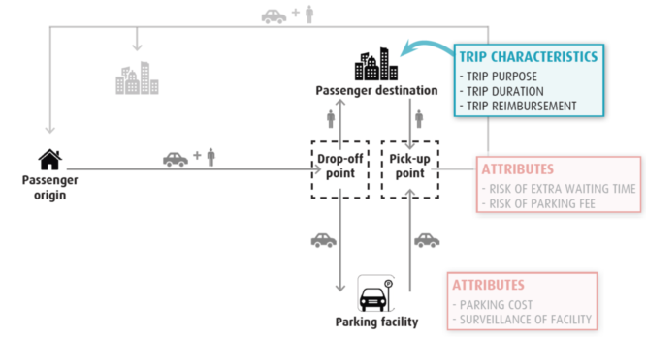
Conceptual framework.
The results of the scenario analysis are visualised in Figure i.2. From the results of the scenario analysis can be concluded that individuals are most sensitive for a change in direct costs, i.e. the ‘parking cost’ at the parking facility and the ‘parking fee’ for temporary parking the highly AV at an on-street parking place near the passenger’s destination.
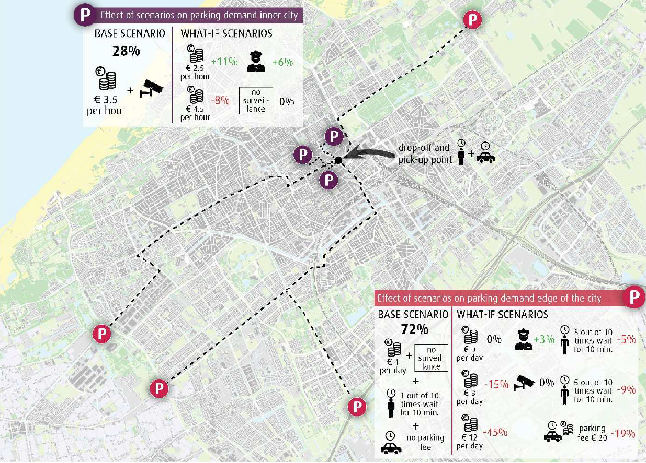
Figure i.2 - The influence of what-if scenarios on the distribution of parking demand.
Directions for parking policies are related to different topics regarding parking regime, parking price and parking capacity. The directions for parking policies are visualised in Figure i.3.
First, in order to reduce the number of on-street parking spaces, it is advised to forbid the parking of highly AVs at on-street parking spaces.
Second, in order to minimize the number of empty vehicle kilometres, it is advised to stimulate short term parking of highly AVs in the inner city and stimulate long term parking of highly AVs at the edge of the city.
Third, it is advised to implement a dynamic pricing strategy for the parking fee that is asked for temporary parking the highly AV at an onstreet parking place near the passenger’s destination, when the highly AV arrives too early.
Fourth, when more parking capacity is needed, it is advised to invest in flexible parking facilities at the edge of the city near distributor roads.
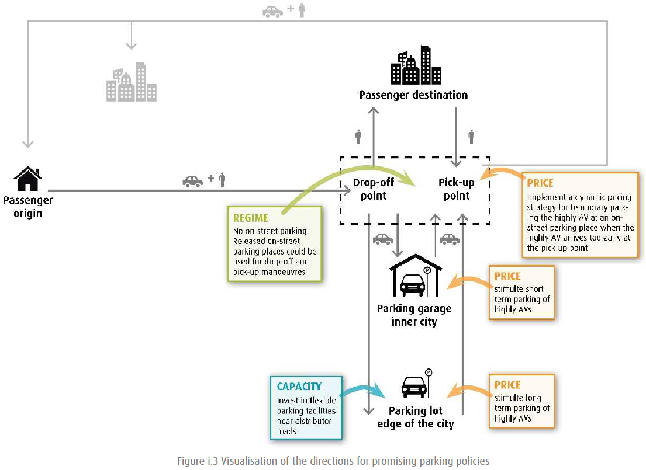
Figure i.3 - Visualisation of the directions for promising parking policies.
The social costs of on-street parking-searching, policy and unpriced externalities, by Michael Mclvor (Vrij Universiteit (VU) Amsterdam)
In a large number of cities around the world, the price for on-street parking is far below the price for off-street parking. This leads to excess demand for on-street parking, high occupancy rates and high search costs for potential parkers, often referred to as cruising.
Cruising can be viewed as an external cost that currently parked drivers impose on other drivers looking for a vacant park, but a cost they do not account for when making decisions about when to depart. If a currently parked driver continues to park, this marginally increases the occupancy rate, and marginally increases arriving drivers’ in-vehicle search and walking time costs.
Zakharenko (2016) shows that the optimal price of parking is one that internalises this marginal external parking cost. Building on Zakharenko, we demonstrate that this cost can be estimated using the parking inflow rate, the occupancy rate and the number of bays in an area.
External parking costs encompass both additional in-vehicle search and walking time costs incurred by arriving drivers. We show that the unpriced marginal externality is the key metric that parking authorities should use to inform their parking policies. We apply this methodology to the city centre of Melbourne, where strict time limits are combined with on-street parking prices that are below short-term off-street parking prices.
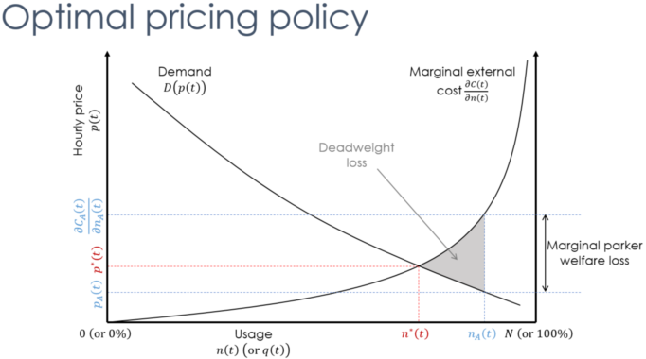
Optimal pricing policy to increase welfare.
We demonstrate that generally parking externalities are low and far below their optimum, so relaxing many of the current parking time limits will increase welfare. Alternatively, on Sundays in many areas parking externalities are high while parking is free, so introducing paid parking will also increase welfare. Similarly on weekdays and Saturdays late in the evening just before restrictions end parking externalities are high, and so extending their hours of operation will also improve welfare.
Know before you go: predicting parking space occupancy by exploiting publicly accessible data, by Robert Boer (Erasmus University Rotterdam (EUR))
In an attempt to guide drivers towards vacant parking spots, currently existing solutions provide real-time parking space availability information. These solutions are far from optimal, as the information disseminated might have already become obsolete by the time of arrival. It would therefore be of great benefit to drivers when parking space availability upon arrival can be predicted in an accurate manner well ahead of time.
Although previous research has attempted to predict parking space by including external variables in predictive models, it falls short in attributing significant attention to the identification of external variables that are capable of improving accuracy obtained from prediction algorithms. Furthermore, prior literature has failed to investigate the impact of extending the time horizon of predictions on the prediction error of the models.
In order to close these gaps in literature, (1) we identify to what extent the inclusion of external, publicly accessible data in the parking space prediction model influences its predictive performance and (2) we assess the effect of extending the forecasting horizon up to 24 hours on the predictive performance of parking space prediction models.
Our research shows that the inclusion of external variables in prediction models for parking space occupancy can significantly improve its performance. Compared to baseline models that only leverage historical occupancy, we are able to reduce error rates with up to 49.15% by including external variables.
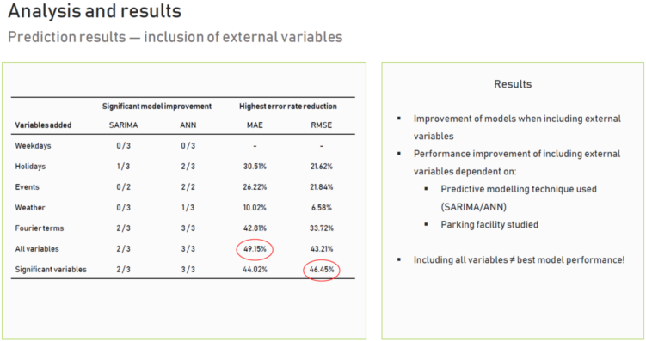
Prediction results
We also found that the inclusion of event information as external variables in Artificial Neural Networks leads to significant forecast improvements, particularly for parking facilities situated in areas where (large-scale) events happen on a regular basis.
And our results show that, although forecast errors increase rapidly for small step ahead predictions, error rates typically converge to a stable and acceptable maximum error rate after predicting six hours ahead of time. This paves the way for informing drivers by disseminating parking space predictions in real time via web-based - or smartphone applications or other media.